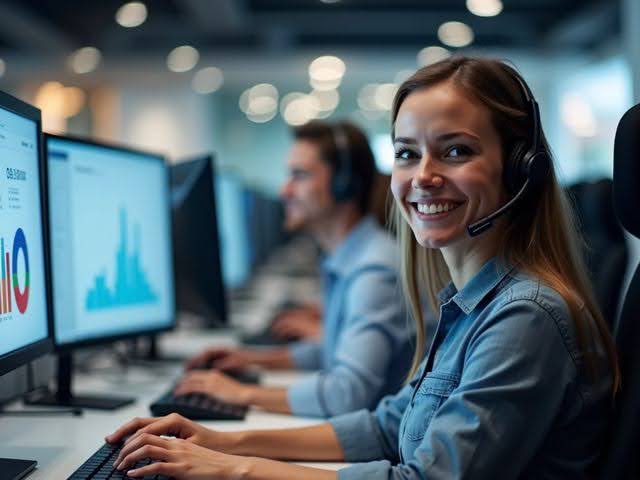
Customer sentiment, intent classification, resolution detection, review triggers, and predictive CSAT models empower CX analysts to decode emotions, uncover reasons for outreach, and improve customer satisfaction. Using AI models, CX analysts can derive powerful insights from vast amounts of data to enhance customer experiences and business outcomes.
The AI Models CX Analysts Need to Know
AI is reshaping customer service—but not all AI models are created equal. For the customer experience (CX) analyst, understanding the mechanics behind these models isn’t just helpful—it’s critical. The difference between meaningful insight and misleading noise often comes down to knowing what’s under the hood.
This guide breaks down the five key AI models powering modern CX analytics. You’ll learn how they work, how to evaluate their effectiveness, and the questions to ask when selecting vendors. Because if you’ve ever stared at a dashboard and thought, “How did they even get this number?”—you deserve a better answer.
Why Knowing AI Models is Not Optional
The flood of customer conversations hitting service teams every day—across voice, chat, email, and social—makes manual analysis impossible. Retailers, for example, manage thousands of daily interactions, each packed with sentiment, feedback, and emerging issues waiting to be spotted.
AI-powered conversation intelligence changes the game. Instead of sampling a few transcripts, analysts can review 100% of interactions to get the real picture of customer experience. These tools help businesses grasp customer behaviors and emotions that shape better engagement strategies.
But the value of these AI models isn’t about replacing people.
The best results come when analysts use AI-generated insights to make faster, smarter decisions, freeing up time for creative problem-solving and strategy.
Consider how retailers use AI for personalized marketing—matching offers to individual preferences. Or how leading financial firms apply predictive analytics to flag fraud before it impacts customers. AI enables brands to deliver smooth, reliable experiences across every touchpoint.
The bottom line? AI doesn’t replace CX analysts—it amplifies them.
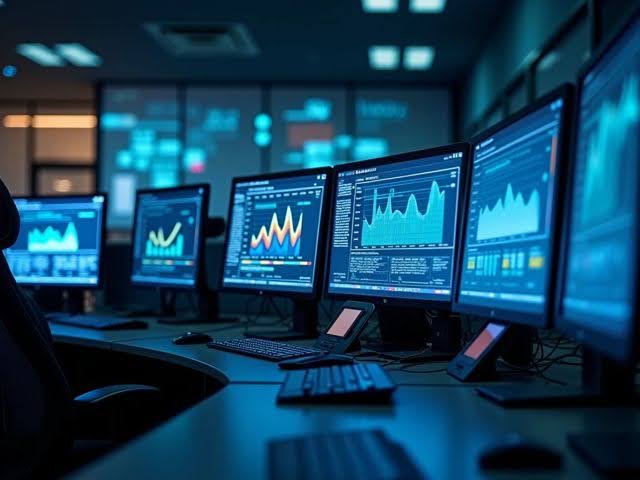
1. Customer Sentiment: Tapping Into How People Really Feel
Sentiment analysis goes beyond simply labeling a conversation “positive” or “negative.” It’s about understanding the emotional trajectory of each interaction:
- Did the customer leave the conversation feeling better or worse?
- Is the frustration aimed at the customer service agent or at the company’s policies or products?
The strongest sentiment AI models use natural language processing (NLP) and machine learning (ML) trained specifically on customer service conversations, since those have a clear purpose and multiple parties interacting to come to a solution. Generic AI models trained on social media posts aren’t enough. Basically, you need to use models trained on the types of data you want to analyze.
How It Works:
Sentiment models like the one used by Loris apply transformer architectures (e.g., BERT, RoBERTa), looking at message-by-message sentiment and scoring on a 5-point scale from very dissatisfied (-2) to very satisfied (+2). These AI models account for conversation elements that keyword based approaches often miss, like polite refusals (e.g. “no, that’s it”, “no thanks”).
Practical Applications:
- Identify churn risks early by tracking negative sentiment spikes across key contact drivers and customer cohorts.
- Evaluate how process changes (like a new onboarding flow) or products (like a new feature launch) are impacting customer experience.
- Analyze customer sentiment to understand whether you have an agent quality issue or if specific policies, products, or other factors outside the agent’s interaction are upsetting customers.
How to Evaluate Sentiment AI Models:
✔️ Was the sentiment model trained on the types of conversations or other data that I care about?
✔️ Does it enable me to track changes in sentiment to understand what’s behind customer reactions?
✔️ Can it distinguish the target of the customer sentiment (i.e. agent-related vs policy-related frustration?)
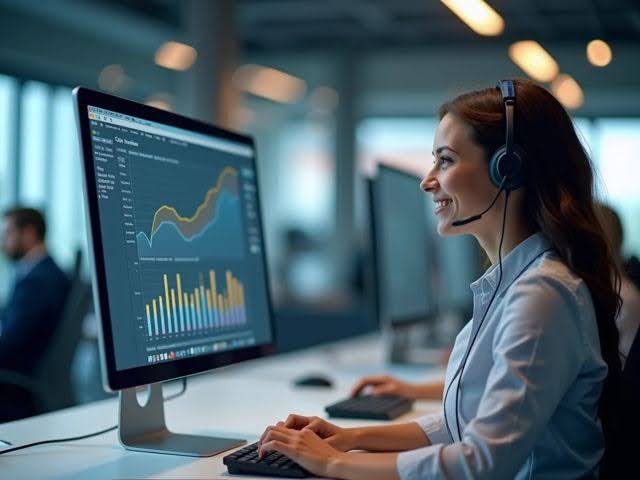
2. Contact Drivers (Intent Classification): Uncovering the Real Reasons for Outreach
Why are customers reaching out? Intent classification models provide the answer—categorizing conversations by issue type (e.g., “Refund Request,” “Shipping Delay,” “Account Lockout”).
How It Works:
Advanced intent models use deep learning-based classifiers like BERT, DIET, or DistilBERT, fine-tuned on thousands of labeled customer interactions. They handle multi-intent detection and understand context far better than legacy keyword-based tagging.
Example:
A retail customer saying, “Still waiting on my refund—it’s been two weeks” may pick up both “Refund Request” and “Shipping Delay” as issue topics, but the one expressed first will be the primary Contact Driver.
Why It Matters:
- Helps product and CX teams prioritize issue fixes.
- Supports smarter staffing—e.g., increasing billing-trained agents when payment dispute volume spikes.
- Enables proactive communication when new issues emerge.
How to Evaluate Intent AI Models:
✔️ Is the model trained on your company’s data to pick up your specific issues?
✔️ Does it support multi-intent detection, so you can see the issues expressed during the interaction?
✔️ How accurately can it detect emerging issues?
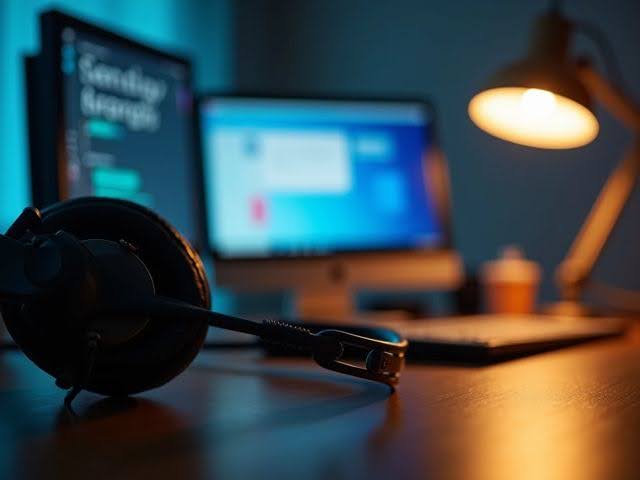
3. Resolution Detection: Measuring If Issues Were Truly Solved
One of the most frustrating customer experiences is needing to contact support multiple times for the same issue. Resolution models help CX teams identify whether a problem was actually solved during the interaction.
How It Works:
Resolution detection combines sequence classification models with dialogue state tracking. These models analyze if the agent provided a clear solution and whether the customer acknowledged the resolution—or if questions remain open.
Example:
A retail refund conversation that ends with “When will I get my money back?” suggests unresolved status that could lead to further follow up, even if the agent thought the case was closed.
Why It Matters:
- Reduces repeat contacts and improves first contact resolution (FCR).
- Focuses QA and coaching on unresolved cases.
- Predicts churn risk tied to failed resolutions.
How to Evaluate Resolution Models:
✔️ Does the model explain unresolved cases clearly?
✔️ Does it use the full conversation, not just the final message?
✔️ Are its outputs validated against repeat contact rates or CSAT?
Learning from Klarna: Exposing the Myths & Facts of AI Agents
Join industry experts as they analyze Klarna’s strategic shift and what this means for broader AI agent adoption.
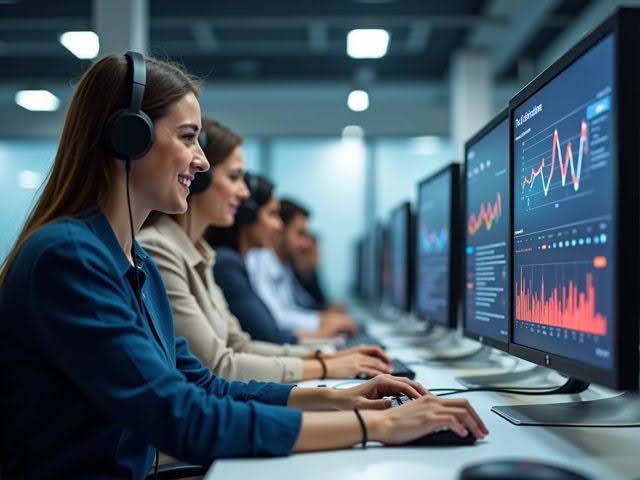
4. Reasons to Review (Red & Green Flags): Highlight the Conversations That Need Attention
Review-triggering AI models help QA and compliance teams focus on the right conversations—those with high stakes or unusual patterns. In fact, many teams create logic that automatically escalates these conversations for review, making sure they get prioritized immediately.
How It Works:
These AI models apply multi-label classification to flag conversations with risk indicators like:
- Compliment (“You’re amazing – thank you!”)
- Profanity (“This is some @#%^$!”)
- Regulatory threats (“This is fraud!”; “I’m going to report you to…!”)
- Customer confusion (“Say that again?”; “Wait, what?”)
- Escalation requests (“I need to talk to a manager…”)
- Repeat complaints (“This is my 2nd time calling about this!”)
These work across every conversation, not just random samples, making sure that threats to you business, whether caused by a poor experience, flawed product, or undertrained agent, is addressed immediately.
Why It Matters:
- Helps ensure quality coverage is targeted and impactful.
- Enables faster intervention on critical customer moments.
- Supports compliance monitoring across industries like finance, healthcare, and eCommerce.
How to Evaluate Review Trigger AI Models:
✔️ Are triggers keyword-based or actual AI models?
✔️ Does the reason the conversation was flagged match what I’m seeing in the conversation?
✔️ How often are there false positives or missed cases?
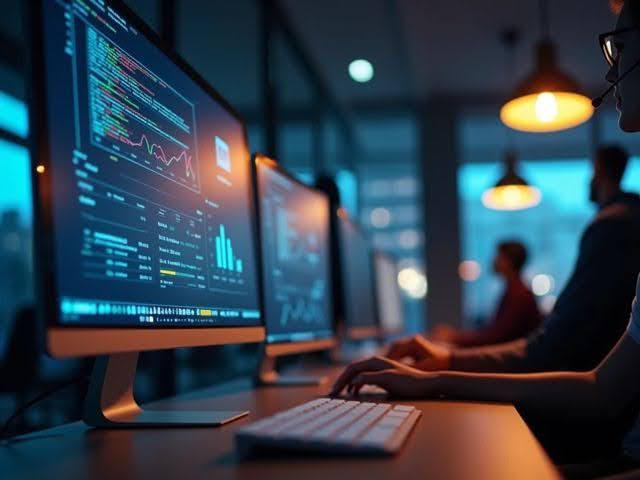
5. Conversation Quality (Predictive CSAT): Measuring Satisfaction Without the Survey
Surveys tend to tell you how the loudest 7-10% of your customers feel. This leaves you with little usable data to make decisions. Predictive CSAT models help fill that gap by predicting the customer satisfaction score for every conversation —no surveys required.
How It Works:
Predictive CSAT models use an ensemble of inputs:
- Sentiment delta (incorporating beginning and ending sentiment)
- Resolution status
- Conversation markers (Customer and Agent interaction behaviors)
The Loris Conversation Quality (CQ) model provides a predictive CSAT that has been proven to correlate with survey-based CSAT.
Why It Matters:
- Highlights potential issues for customers who never filled out a survey.
- Tracks CX performance using a consistent benchmark across all agents, channels, and issue types.
- Provides a leading indicator for churn or satisfaction problems.
How to Evaluate Predictive CSAT AI Models:
✔️ Does the model have evidence of a strong correlation to actual CSAT?
✔️ Does the score reflect what’s actually happening in the conversation?
✔️ Is the model proven overtime or is it something theoretical that was just developed?
The Real Challenge: Using AI Models to Turn Overload to Action
CX analysts often run into obstacles like:
- Sorting through thousands of chat and voice transcripts to spot trends or compliance issues
- Making sure agent evaluations are fair, consistent, and actually useful—not just a formality
- Pulling meaningful, real-time insights from every conversation, instead of relying on random samples
AI models now automatically score every conversation using customizable scorecards, quickly highlighting where agents excel and where they can improve. For example, using metrics like average handle time (AHT), agent performance scores, and CSAT, Quality Assurance tools can identify which agents need coaching and which processes are causing friction for customers.
The real leap forward comes when you bring together multiple perspectives on a single platform—with Customer Insights giving you voice-of-the-customer analytics insight and Quality Assurance providing the virtual and human side of the conversation. This integrated approach gives CX leaders a clear, broad view, helping them move from reacting to problems to designing better customer experiences.
From Conversation Data to Business Impact
The best CX analysts don’t just report on the past—they shape what happens next. By understanding these five models, analysts can unlock insights that drive measurable business outcomes:
- Automate for Scale: Let these specially designed AI models review every customer interaction, not just a sample. Companies like Calendly have cut costs by 23% using conversation intelligence to surface actionable insights across all channels. With this kind of coverage, you can spot issues before they spread.
- Make Feedback Useful: Use AI to summarize conversations and spot shifts in sentiment as they happen. Tools such as The Gist condense long transcripts into clear summaries, helping product and operations teams focus on fixes that matter to customers.
- Measure What Counts: Don’t settle for surface metrics. Combine AI-driven conversation scores with business KPIs. Using Customer Insights to monitor every interaction helps companies spot churn risks and product pain points faster—leading to real improvements in loyalty and retention.
- Keep Improving: Don’t let your AI models get outdated. Provide feedback to continuously update your AI, making future insights sharper and more relevant. This ongoing improvement helps you stay ahead of customer expectations.
How AI Models Help Analysts Build Trust and Accountability
AI models work well with solid data and strong processes behind them. High-quality, structured data as fuel for human decision-making makes sure AI insights are reliable and ready to act on.
CX analysts and leaders who coordinate these AI models don’t just understand their customers—they anticipate needs, adapt quickly, and prove ROI with real numbers. With the right AI platform, conversation data becomes a strategic asset, turning the call center from a cost center into a competitive advantage.
Questions to Ask When Evaluating AI Vendors
Question | Why It Matters |
Were the AI models trained on the types of data I’m trying to understand? | Ensures relevance and accuracy for your use case. |
How are outputs used for feedback? | Builds trust and allows for continuous learning. |
How do you measure AI model performance? | Provides insight into whether you or the vendor will maintain the AI models. |
Does it cover 100% of conversations? | Avoids bias from survey-only feedback or random sampling. |
How have clients tied AI outputs to business KPIs? | Shows clear ROI and justifies investment. |
The Bottom Line: Smarter AI Models, Stronger CX Analytics Team
The goal isn’t just to use AI—it’s to use it well. These five AI models give every CX analyst the power to understand customer needs at scale, improve agent performance, and create more consistent, human-centered experiences.
When data quality is high and human expertise guides the process, conversation intelligence becomes your secret weapon for loyalty, retention, and operational success.