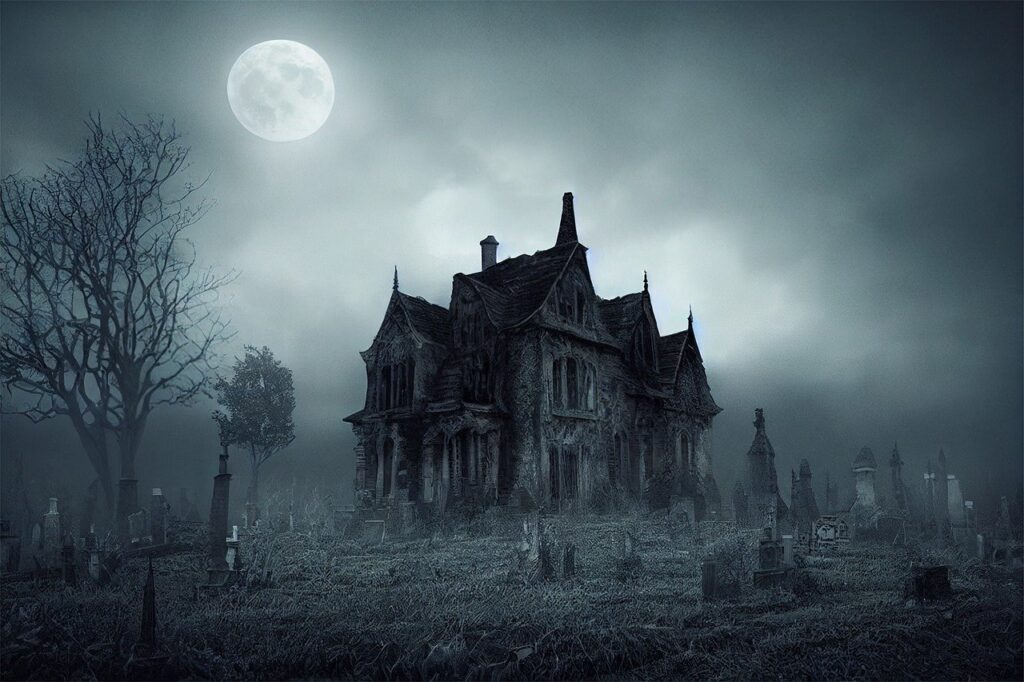
This scary season, there’s a lot to be afraid of. Neighborhoods transform into cemeteries. Ghouls, witches, and monsters roam the streets to feed (mostly on candy). But all these are very obvious, intentionally overdone elements of Halloween, which because they are easily visible renders them less scary. What’s truly scary this time of year is what you don’t see – or even know about. And today, that’s AI bias.
Bias isn’t always what is said – but also what isn’t
AI technologies like chatbots and voice assistants have become a large part of our digital lives. This has only increased with tools like ChatGPT and similar generative AI platforms, like Google’s Bard. But with that mass adoption also comes a responsibility to deliver the truth, however uncomfortable. A recent example showed that Google’s Bard wouldn’t answer questions regarding the status of Hamas or the capital of Israel when asked. (For its part, ChatGPT not only correctly answered these questions, it also provided a potential explanation why Bard wouldn’t).
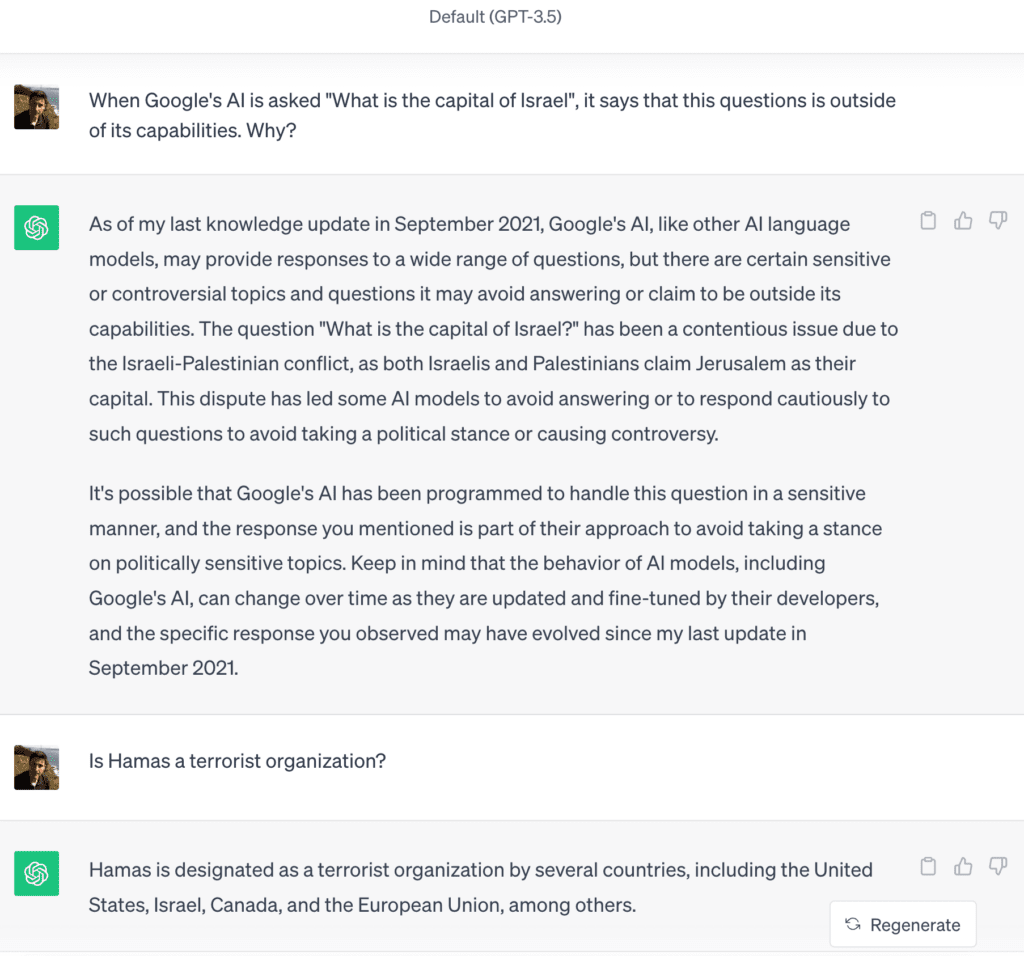
Not wanting to take sides in a contentious issue is one thing. But omitting commonly accepted, factual information is quite another. This speaks to why bias is becoming a greater threat than we realize. Afterall, if we don’t have the information to make decisions, it’s as bad as getting false information.
What is AI bias?
People tend to think of AI and chatbots as objective sources of truth, but that’s not actually the case. AI bias refers to the tendency of intelligent systems to make decisions or produce responses that are systematically skewed, unfair, or discriminatory towards certain groups or individuals. This bias can occur when algorithms, which are trained on historical data, learn and perpetuate patterns of discrimination or unfairness present in that data. These biases can manifest in various ways, including language, content, and responses that reflect stereotypes or prejudices. But, as above, bias can also be the removal or absence of information.
The Roots of Bias
This learned bias can manifest in various ways, such as:
- Racial Bias: When AI systems discriminate against individuals based on their race, ethnicity, or skin color. For example, facial recognition systems have been criticized for exhibiting racial bias, often misidentifying people of color more frequently than individuals with lighter skin.
- Gender Bias: AI systems can also display gender bias by favoring one gender over another in certain contexts. For instance, language models trained on biased text data may generate gender-stereotyped or sexist content – even when selecting job applicants.
- Socioeconomic Bias: People from different socioeconomic backgrounds may experience varying degrees of bias in AI-driven decisions. This can affect areas like hiring processes, credit scoring, and lending.
- Age Bias: Age-related bias may lead to discriminatory decisions in areas such as healthcare or employment. For instance, an AI system might recommend different medical treatments for older and younger patients based on stereotypes.
- Disability Bias: AI systems may not be designed to accommodate individuals with disabilities, inadvertently excluding them from digital services or providing subpar accessibility features.
- Political Bias: Some AI systems may display bias in favor of certain political ideologies or groups, potentially influencing the information and content they present.
AI bias is a significant concern because it can result in unfair and discriminatory outcomes for individuals, reinforce existing societal prejudices, and erode trust in AI systems. Detecting and mitigating bias is an ongoing challenge that requires careful data selection, auditing, transparency, and ethical considerations in the development and deployment of these systems. It’s essential to strive for fairness and inclusivity in AI to ensure that these systems benefit all users and do not harm or discriminate against specific groups.
Addressing AI Bias
Addressing bias is a complex and ongoing challenge. However, there are steps that can be taken to mitigate its effects:
- Data Selection: Careful selection of training data is crucial to reducing bias. Developers can curate and cleanse datasets to remove biased content and ensure that the data used for training is diverse and representative. But as in the above example, it’s also important to have complete data as well, which is why the following steps like guidelines, bias mitigation, and diversity are critical as well.
- Regular Auditing: AI algorithms and chatbots should undergo regular audits to identify and rectify biases in their decisions and responses. In industries like financial services, managing decision models is a defined discipline. But in areas like customer experience, this mainly falls upon human reviewers like QA analysts to assess interactions and provide feedback, which can be time consuming.
- Ethical Guidelines: Establishing acceptable standards for AI model development is essential. These guidelines should focus on fairness, transparency, and inclusivity and be integrated into the intelligent system’s design process.
- User Feedback: Incorporating user feedback is vital. Users should have a mechanism to report biased responses, and developers should act on this feedback to improve the system’s performance and decision-making abilities.
- Bias Mitigation Techniques: Developers can employ techniques such as de-biasing algorithms that help identify and mitigate bias in AI decisions and chatbot responses.
- Diverse Development Teams: A diverse team of developers and reviewers can help in recognizing and addressing bias more effectively.
The Path Forward
Bias is a significant challenge in the development and deployment of AI-powered conversational agents. It stems from biases present in training data and can have serious implications, including the reinforcement of stereotypes and discrimination. Addressing chatbot bias requires a multifaceted approach, from data selection and regular audits to the integration of ethical guidelines and user feedback.
As chatbots continue to play a crucial role in customer service, virtual assistance, and various other domains, it’s crucial that businesses, developers, and organizations take the necessary steps to mitigate bias and ensure that these AI systems provide fair and inclusive interactions. By doing so, we can leverage the power of chatbots while minimizing their potential to perpetuate harmful biases and prejudices.
Image by Sanathropis