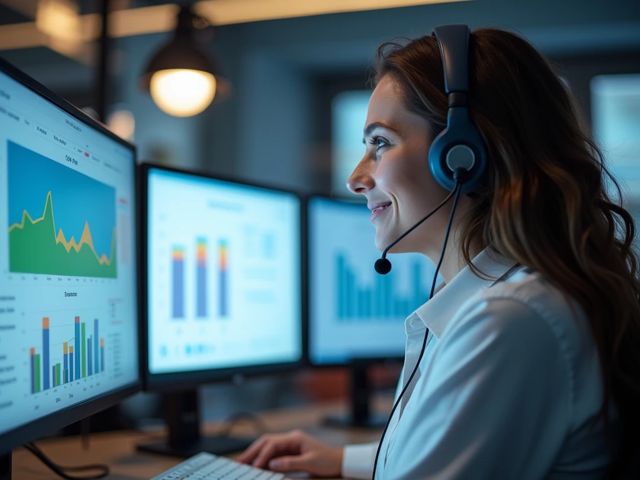
Measuring AI agent performance hinges on metrics like resolution rate, sentiment trajectory, and emotional triggers rather than outdated human-centric scorecards. These data-driven insights offer a holistic view of conversation quality and customer experience.
Why Traditional Scorecards Miss the Mark in AI Agent Performance Measurement
Imagine this: A leading ecommerce brand launches AI agents in its call center, expecting round-the-clock efficiency and instant answers. A few weeks later, the operations team sits down to review performance—but they’re still using the same old QA scorecards built for human reps. The outcome? Confusion, frustration, and a lot of unanswered questions about what’s actually working.
Why Traditional QA Doesn’t Work for AI
Supporters of traditional QA processes often say that scorecards and manual reviews maintain high quality. That would be true, if you could provide coverage for a majority of your agents and conversations. But when you’re only looking at less than 3% of all available interactions, what kind of quality are you really getting?
Traditional methods depend on subjective judgments, small samples, and criteria like empathy or building rapport, which made sense for people but don’t line up with how AI should be measured. For example, a QA analyst might listen to 5 out of 1,000 calls per agent each month. That’s less than 1%—nowhere near enough to spot trends or issues in an AI that handles thousands of interactions every day.
In retail and fintech, this mismatch is even more obvious. AI agents don’t get flustered or go off-script, so “soft skills” metrics don’t really apply. The potential for AI agents is there; organizations are seeing that AI can resolve up to half of all routine customer questions. But using the same human-based approach misses out on important insights like consistency, response speed, and intent recognition.
The Problem With Sample-Based Reviews
Those skeptical of change tend to point out that sampling is efficient and keeps things “fair”. With AI, though, the sample size issue gets even bigger. AI agents handle huge volumes—think 10,000+ chats a week in a busy ecommerce setting. Chances are, you’ll miss patterns like repeated misunderstandings around payments or compliance slip-ups in sensitive financial conversations.
Manual reviews bring in another problem: subjectivity. When evaluators aren’t trained on how AI agents behave, scoring gets inconsistent. This can lead to misleading assessments and missed chances to improve.
Time for a New Playbook
Old-school methods just don’t work for evaluating AI agent performance. When 70% of customer experience leaders admit AI’s impact is “difficult to measure” with current tools, it’s a clear sign that the old playbook needs an overhaul. Teams ahead of the curve are moving toward objective, data-driven metrics that match how AI really works in support roles, rather than sticking with subjective, human-focused scorecards.
McKinsey analysts believe that, in the next 12-18 months, AI will resolve up to half of all routine customer questions, underscoring the shift needed from human-based standards to metrics capturing AI-specific insights.
Bottom line: If you’re still grading your AI agent performance the same as human agent performance, you’re using the wrong test. Rethinking what “quality” means for AI-driven call center software is the only way to unlock the benefits you set out to achieve.
The Power of Full-Context Data: Moving Beyond Guesswork
Call centers have long faced a classic gap: you can only manage what you can measure. And for years, that meant reviewing a tiny fraction of support conversations—often less than 2%. Teams have been left in the dark about what really happened in the other 98% of interactions. With the emergence of AI agents handling more and more customer touchpoints, that gap can only be expected to grow. The outcome? An incomplete, sometimes misleading view of customer experience and support quality.
The Pitfalls of Partial Data
When only a small portion of your overall AI agent-led conversations get reviewed, important trends can slip through the cracks. For instance, a retail brand might notice that 85% of sampled chats ended with a resolution, but what about the conversations no one checked? Did those customers leave satisfied, or did they give up and abandon their carts?
Sampling misses subtle but meaningful patterns, like a sudden rise in negative sentiment after a policy update, or repeated confusion around a new feature. These blind spots can lead to lost sales, higher churn, and damage to brand reputation.
Data-Driven Insights: Assessing 100% of Conversations
Advanced analytics platforms focused on AI Agent performance now make it possible to review every single AI agent-led conversation. That means brands can stop guessing and start acting on solid data. Here’s what that looks like:
- Resolution Rate: Instead of guessing if issues are resolved – or trusting the AI agent provider who charges per resolution – get a clear accounting of successful outcomes across all conversations. In fintech, this could mean tracking how many customers actually completed a transaction after chatting with an AI agent, not just those from a small sample.
- Sentiment Analysis: By applying sentiment analysis to every exchange, you can follow the emotional journey of each customer—from initial frustration to relief, or ongoing confusion. This approach digs deeper than “was it resolved?” and asks “how did the customer feel throughout the process?” This can help you answer the question
- Review Reasons: Data-driven tools can flag moments when customers express confusion, anger, compliments, or other emotional triggers. If confusion keeps popping up whenever an eCommerce chatbot explains a return policy, you know exactly where to step in and make improvements – either in how that policy is explained or who is handling those issues.
The Impact: Smarter Decisions, Better Outcomes
With access to the full story, customer experience leaders can spot and fix issues before they grow into bigger problems. For example, brands that review all conversations have seen up to a 25% increase in resolution rates and fewer customer complaints.
More complete data opens the door to tailored agent retraining and smarter AI agent performance updates. If analysis shows customers lose trust when asked to verify their identity twice in one session, you can adjust the workflow and test the results—no more guessing.
Key Metrics for Conversation Quality
Metric | What It Reveals
|
Resolution Rate | Percentage of issues truly solved, excluding those transferred to a human agent or abandoned by the customer |
Sentiment Trajectory | The change in the customer’s emotional temperature from their initial message, throughout the conversation, and at the end. |
Review Reasons | Key moments of confusion, frustration, or delight that warrant attention, remediation, or review. |
Switching from partial sampling to complete, data-driven insights changes how brands understand and improve AI agent performance. It’s not just about tracking if a problem gets fixed—you’re seeing how every customer feels along the way, and that’s what builds loyalty in a crowded market.
Matching the Right Issue to the Right Channel
When it comes to delivering seamless customer support, getting contact routing right can make all the difference between a loyal brand advocate and a frustrated ex-customer. Modern call center software, powered by tools like Loris, brings data-driven clarity to a classic challenge: Which issues should go to AI agents, and which need a human touch?
AI Agents vs. Human Agents: Matching the Right Help to the Right Problem
AI agents shine when handling repetitive, low-complexity requests – such as order status updates, password resets, or account balance checks. These interactions follow predictable patterns and carry little risk, making them ideal for automation. Customer conversations packed with confusion, frustration, or hints of potential churn call for the empathy and judgment of a human agent.
Understanding Customer Sentiment
Sentiment analysis tools do more than spot angry customers—they pick up on subtle cues throughout the conversation. For instance, if you notice a decline in customer sentiment for AI agent conversations for a specific topic, it could be an indicator that AI agents aren’t the best ones to handle that topic. Sending high-stakes, low-sentiment interactions to human agents who can do things like build rapport and empathize can reduce customer frustration and control churn risk.
Real-World Results: Efficiency and Satisfaction
Here’s how this works in two everyday contact center scenarios:
- A customer asks for a shipping update. The AI agent pulls tracking info, resolving the initial request quickly. If there’s an issue with the shipping timeline or some unforeseen delay, the AI agent can pass the customer to the human agent to focus on these trickier issues, but otherwise the AI agent handles it all.
- A customer is upset about a delayed refund and hints at leaving for a competitor. This issue is known as complex, so the AI agent passes the customer to a skilled human agent. The agent listens, offers a goodwill credit, and keeps the customer from leaving.
Routing Issues for Better Results
Smart contact routing – powered by real-time customer sentiment and issue complexity – means automation isn’t just about getting people off the line, but about giving the right experience at the right moment. AI agents take care of routine, predictable, and low-risk tasks. Human agents step in when relationships are at stake.
Brands that combine automation with real human understanding—using data to connect the right issue to the right channel—not only improve performance metrics. They create trust, loyalty, and a reputation for customer care that automation alone can’t match.
Taking a Data-Driven Approach to AI Agent Performance 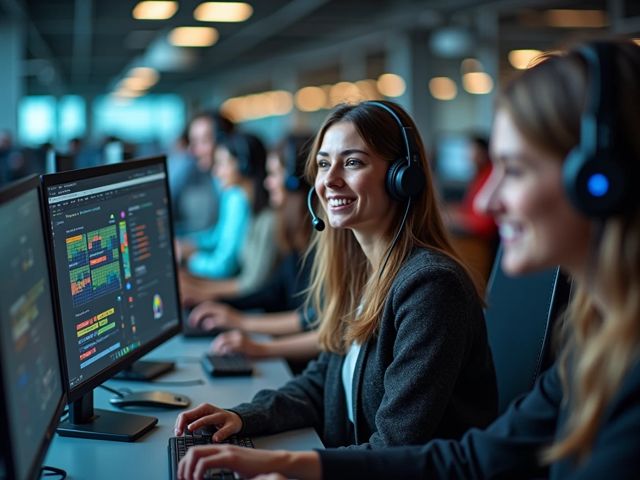
Conversation analytics – tools that break down what’s actually happening between customers and agents – can improve customer support and deliver measurable business results. Using this conversation data, you can not only identify, but also breakdown which signals highlight conversations appropriate for AI agents and the others for human agents. Here’s some practical examples of putting this data to work:
Company Type | Issue | Action | Outcome
|
eCommerce Retailer | AI agent order status flow is leading to customer frustration | Rewrite script and add a clarifying follow-up to ensure issue understanding | Reduction in repeat contacts and increase in customer satisfaction |
Fintech | Consistently low sentiment for specific account management issues | Train AI agent to hand off complex cases to humans | Reduce escalations and churn risk |
Health & Wellness Brand | AI agent product recommendations at risk of violating compliance policies | Create more specific guardrails around responses and route to human agent for additional advice | Increase in customer satisfaction and reduction in compliance risk |
In each of these examples, brands can skip the guesswork and use available data to decide which interactions to automate, which scripts to tweak, and where human empathy is most important. The payoff: higher CSAT (Customer Satisfaction) scores, lower operating costs, and a smoother experience for both customers and support teams.
Better AI Agent Performance Starts With the Right Metrics
The pressure is on: according to Box, 80% of customer experience leaders say quantifiable business impact matters most when evaluating AI agent performance, yet just 34% feel confident their current automation investment is delivering on that promise.
Counting contained or deflected tickets is easy, but those numbers alone don’t show whether automation is actually improving customer experience metrics like CSAT, CQ (Conversation Quality), first contact resolution, or customer lifetime value. Metrics must connect automation investment directly to customer and business outcomes.
AI Agent Performance Optimization: What Moves the Needle
- Quality over quantity: Looking only at the number of automated interactions misses the point. Focus instead on metrics like intent accuracy, escalation prevention rate, and reduced average handle time. For example, brands using Loris have seen both reduction in handle times and a measurable lift in customer retention.
- Customer-centric metrics: AI should do more than just speed things up. Track Voice of the Customer (VoC) insights across every conversation with tools like Customer Insights to see how automation affects satisfaction, loyalty, and even revenue.
- Feedback loops: The most effective AI agent optimization strategies use real-time quality intelligence. With AI Agent Insights and Quality Assurance, support leaders can spot breakdowns and keep training both bots and humans for better results.
Data-Driven Automation in Action
Take a fintech company automating password resets, billing questions, and fraud alerts. On paper, 65% of these tickets get resolved without human help—a win at first glance. But if 40% of those customers call back or leave negative feedback, the picture changes. Loris AI Platform highlights not just how many issues are resolved, but whether those resolutions actually boost NPS (Net Promoter Score) or lower churn.
Turn Insights into Action
Smarter automation isn’t about pushing automation while sacrificing experience. It’s about making sure every automated interaction creates real, measurable business impact. Customer-focused brands can optimize their AI investment by using AI Agent Insights to fine-tune their support workflows, spot hidden friction, and get the most out of every automation dollar.
Want to see what’s possible beyond surface-level stats? Let Loris show you how the right customer experience metrics can turn your automation investment into a real advantage.