First, I’ll give credit where credit is due. There was a fantastic webinar from Gartner last week called “Stop Today’s QA; Elevate to Quality Intelligence (QI)”. So I encourage you to watch the webinar, because a) this isn’t going to be a summary of someone else’s work and b) it has a ton of great points I’m not going to discuss here.
But what I am going to discuss is the idea that maybe this isn’t an evolution of QA, but that we’ve been thinking about Quality Assurance (QA) all wrong.
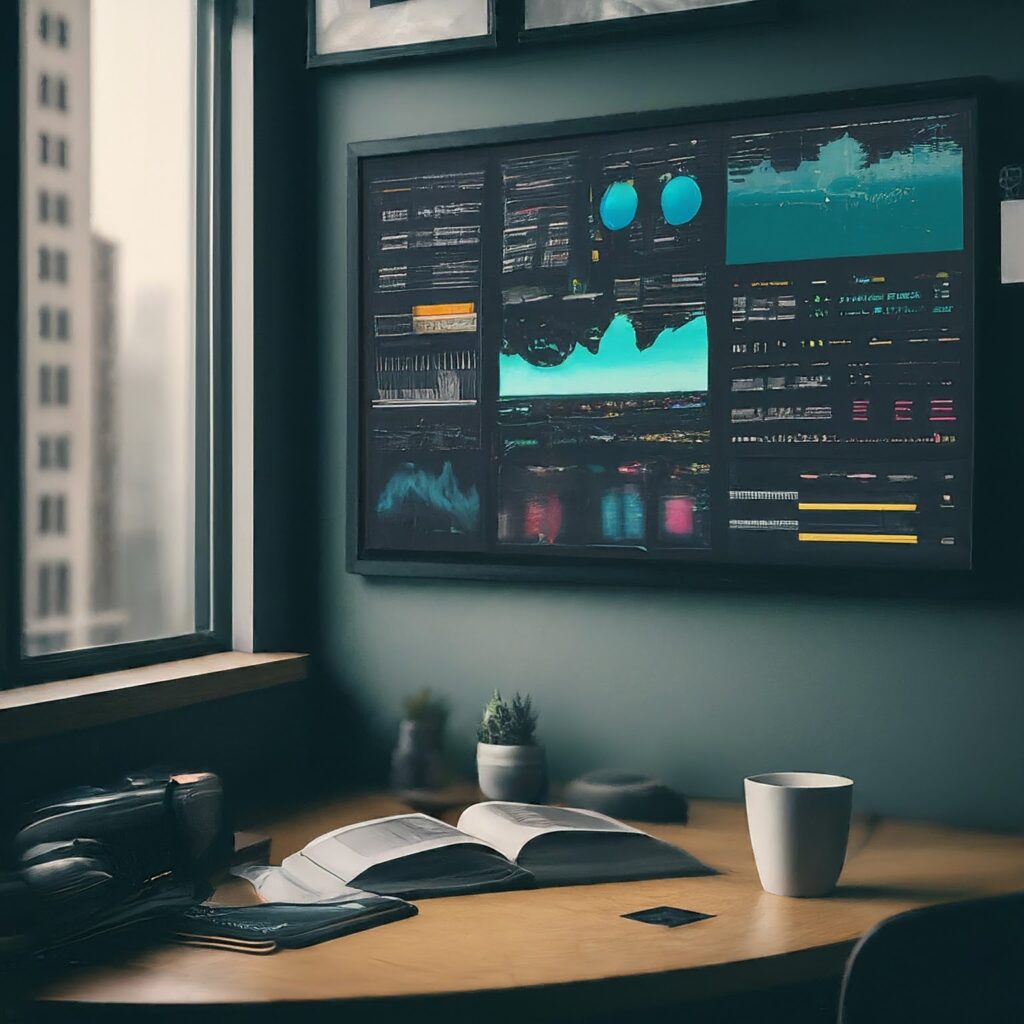
The Limitations of Quality Assurance
For years, QA programs have been the primary method for evaluating customer service performance and ensuring agents are doing and saying the right things. But it’s a lot of work and it’s not humanly possible to assess every conversation. So, in an attempt to be somewhat diligent, organizations randomly selected 2-3% of all conversations for review. If this seems a bit archaic nowadays just keep in mind that even some seemingly modern QA platforms still operate in this way.
The obvious drawback of this approach is that it takes a while to randomly stumble upon an issue, unless a customer service rep is blatantly going against your policies on every single call, chat or email. In addition, the workload this creates for Quality Assurance analysts doing these reviews can be large, meaning a conversation happening right now may not come across their desk for a few weeks – if ever.
The last main issue is the scorecard, which has a list of items upon which the agent is graded. Not only can the questions and scoring criteria be vague or open ended, the assessment itself can be subjective. In many cases, scorecards are tied to agent performance and compensation so poor scores can be contested leading to a lot of back and forth. And once the agent agrees to the scorecard and is coached on it, the scorecard doesn’t have much use.
AutoQA, we hardly knew ye
As AI became more and more of the default approach over the last two years, existing players in the QA space took notice. And as is typical for the first wave of adoption of a new technology, from PCs to Cloud solutions, the initial use case was to add AI to this traditional QA process.
I like to call this the “faster horse” approach.
Instead of trying to leapfrog existing methods, AI was incorporated into them, creating things like AutoQA, which is essentially going through a traditional QA process with pieces of it automated. AutoQA doesn’t try to reimagine what QA should be – or even if QA should be done. AutoQA takes pieces of data to try and select better conversations instead of random selection. And it takes your scorecard and autocompletes as much of it as it can with confidence, which turns out to be 30-40% of it. While this is a time saving, the AutoQA label makes this outcome a bit underwhelming and there are still a host of issues in terms of tying to human and AI elements together. But this isn’t even the primary issue with QA.
The main problem with QA is that it’s main value isn’t QA
Many organizations we talk to have a QA process, but their goal is to use QA as a means to better understand their customers, such as uncover recurring inquiries, spot emerging issues, and pinpoint where things are broken in the customer experience. Basically, they want to use QA to gather Voice of the Customer (VOC) insight. While it seems like a QA analyst is the best fit for this, since they are reviewing conversations, the process isn’t set up for VOC. Their main objective is to measure agent performance, so all of these customer insights are happening as a byproduct.
Many companies find themselves in a position where they’re trying to retrofit QA data to serve as VOC insights – a purpose for which it wasn’t designed. This approach is inefficient and often fails to capture the true essence of customer sentiment and experience.
Gartner makes this point as well, calling is a misalignment, but it’s really about rethinking the order of operations. Instead of using QA to find interesting customer feedback, you should think about using customer feedback to understand if and what to QA. That way you have the broader insight to be more focused in your QA efforts, as well as have a direct line between what they’re telling you and your VOC insights.
The Rise of Quality Intelligence (QI)
Whether you call it an evolution or a reinvention, Quality Intelligence (QI) is the term used to describe this holistic approach, combining customer, customer experience, and quality assurance data to see the big picture. While these have been traditionally siloed into their respective departments or operations, bringing them together allows organizations to make decisions about what to focus on instead of arbitrary sampling of agent interactions.
By starting with this more complete data set, you not only have the ability to determine where to apply things like agent performance reviews or coaching – you also have a faster means of understanding critical customer issues. Using existing QA processes or customer surveys to uncover real customer issues takes time, effort, and substantial analysis. This synthesis of data from the customer, agent, and interaction perspectives gives you this insight almost instantly, so you can spend time on addressing customer issues instead of looking for them.
How we’re thinking about Quality Intelligence at Loris
Following industry trends is a great way to understand what’s happening. But in this case, I think we’re leading the way. This idea of starting with the insights directly from customer conversations to determine where and how to address problems is one we have been advocating for years. And whether you call it quality intelligence, voice of the customer, agent insight, or something else, it’s exactly the kind of shift that AI is not only driving but enabling organizations to make. Here’s how we’re applying this idea today:
1. Direct line between customers and VOC Insights: We analyze all customer conversations capturing authentic customer sentiment, contact drivers, and hundreds of other data points so you have a complete picture of what’s happening and what you can do about it quickly.
2. Insight from the interactions themselves: Loris identifies patterns in customer interactions, highlighting areas of friction in the customer journey. It also correlates this with agent actions, to help you understand cause and effect. Having this level of detail can do everything from spotting the need for more agent training to opportunities for self-service, automation, and proactive communication.
3. Targeted QA, as needed: Starting with agent insight, you can then pinpoint where QA and coaching efforts are most needed, making the process more efficient and effective. It also helps you utilize analysts for customer advocacy and voice of the customer analysis, which has a greater impact on the customer experience than agent performance alone.
4. Holistic view of CX: By combining these elements, you have a comprehensive understanding of your customer experience and can make the right decisions quickly, instead of using multiple methods to get bits and pieces of insight. Conversations, if taken from all your channels, can provide a more reliable source of insight than QA sampling and customer surveys, which both suffer from low participation rates.
Embracing the Future of Customer Insight
The shift from traditional Quality Assurance to Quality Intelligence represents a significant evolution not only in how businesses understand and respond to their customers, but also how they perform certain functions. And often, it’s the operational side that takes the most effort to change. But QA really can’t keep going on the way it is.
In this new era of CX and AI, the ability to capture, analyze, and act on comprehensive customer data is already a key differentiator. Quality Intelligence is one of the areas that AI is reinventing, but it’s hardly the last. The one thing that is clear is that the organizations that thrive will be those that can effectively listen to their customers, understand their needs, and respond with agility and precision.