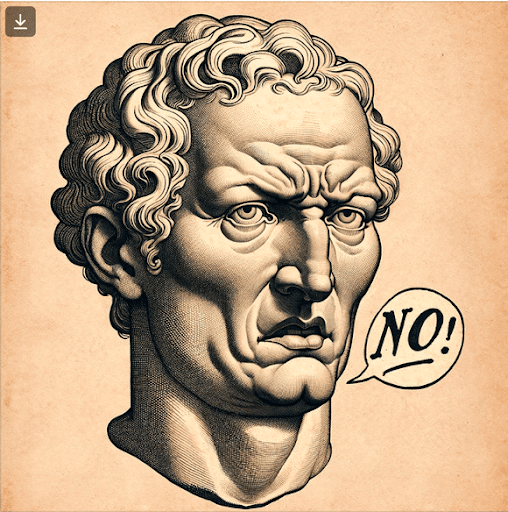
There are no dumb questions.
But… “could you summarize this for me?” gets close.
Very close.
The short answer is no, I cannot! Not without knowing who you are and what you want to use the summary for.
Why so agitated, you ask? Because we have been thinking about summarization all wrong.
How we got started with summarization
Text summarization was traditionally defined as the process of distilling the most important information from a source text into a shortened version, with the goal of creating a concise and coherent version of the original text while retaining its key points and overall meaning.
There is no one summary!
The problem is that there is no one perfect summarization of anything.
What you summarize matters. Who you summarize for matters. And, most importantly, why you summarize matters.
The nuance of summarization in customer service and support
If you are reading this post, you are interested in summarizing customer support conversations. This will be our focus and we will ignore other types of content.
A trainer who wants to coach agents on de-escalation techniques is interested in the back-and-forth part of a conversation that led to the customer being upset. They are less interested in the details of the customer’s issue.
The priorities are exactly reversed for a product manager who wants to know and be aware of emerging issues. They would love a detailed description of the issue the customer reported and are less concerned with the agent’s tone.
A CX manager who wants to offer some special accommodation to customers whose support interactions did not end in a satisfactory way is mostly interested in the resolution status and satisfaction parts of conversations.
Why is this new? What changed about summarization?
Most papers on summarization dealt with summarizing news articles, movie reviews and similar content. Typically, the “who” and the “why” were not stated. Evaluation of produced summaries was done by comparing them to ones that humans create. No specialized knowledge was required of these human summarizers.
Why was the question of whether a summary is fit for a purpose overlooked? Because until recently, the challenge was getting a machine to output a summary that reads fluently and makes some sense. Researchers were developing purpose-built algorithms and models for the task of summarization.
And then came LLMs.
They solved the problem of ok-sounding summaries without being developed solely for this purpose. Solving specific problems in Natural Language Processing without trying too hard is a pattern with LLMs. Just ask the people who wrote their entire PhD dissertations on Coreference Resolution (basically, figuring out that “she” in the text “Ana loves reading. She reads 5 books a month” refers to Ana).
The question of whether a summary is useful for a specific person for achieving a specific goal was like the next hill that you don’t see until you reach the summit of the hill that is in front of you.
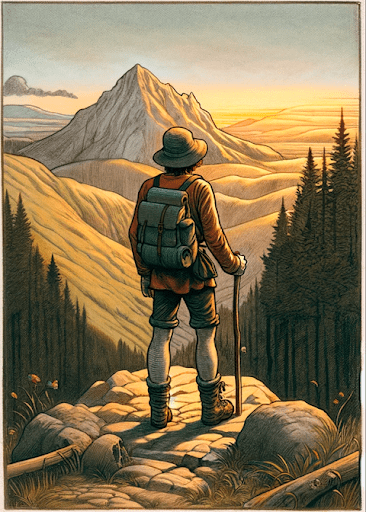
Now, a paper is declaring “Summarization is (Almost) Dead” (meaning that LLMs are doing a pretty good job at it), and I start seeing papers that deal with user preferences for summaries (e.g., here). I believe the interest in more concrete summaries fit for a purpose will grow.
Summarization as an AI assistant briefing
I propose a new way to think about summarization. Currently, AI is at its best when it acts as an assistant to a human. When I say I want a summary of a conversation, I would love for a capable, knowledgeable expert to read the conversation for me and give me a briefing. Getting a competent briefing saves me time and attention and helps to achieve a goal, perform a task, respond to a situation. An AI assistant is that expert.
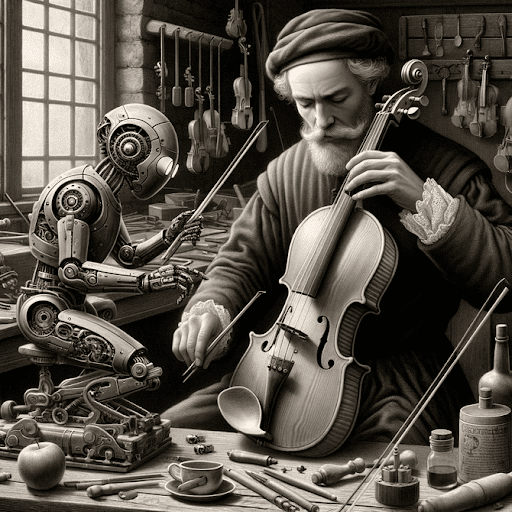
In order to get good answers, you must ask good questions. What should you know about this conversation in order to achieve your goal? A good conversation summary is question-driven. Your AI assistant must have some expertise in the field of customer support in order to answer these questions well.
Question-driven summarization of customer support conversations
Here are possible questions that a summary can answer. A product manager, a trainer, a CX manager from earlier in the post can ask some of them:
- What was the reason the customer contacted support?
- Did the agent (or agents) offer a clear and actionable solution to the customer’s problem, addressing all of the customer’s concerns and questions related to the issue?
- Was the customer satisfied with the solution that the agent offered?
- Did the customer ask to escalate and talk to someone else (manager, supervisor, a different agent)?
- Did the agent accommodate the customer’s request to escalate?
- How did the customer feel about the conversation by its end?
- Is there any followup the agent is required to do after the conversation?
- Do we predict the customer issue as resolved?
A conversation summary that answers these questions may look like this:
The customer contacted support because they were experiencing an issue with their payment not going through. The system was repeatedly showing an “insufficient funds” error for their payment, even though the customer claimed that all of their cards had sufficient funds.
The agent did not offer a clear and actionable solution to the customer’s problem. While the agent explained that the payment had already gone through with a different card, they did not address the customer’s request to fix the system issue causing payment errors, which was the main concern raised by the customer.
The customer expressed frustration with the system issue, as they used an emoji indicating frustration (🤦🏽♀️) in their response, indicating their dissatisfaction with the resolution provided by the agent.
The customer’s issue is not predicted as resolved due to the agent not addressing the system issue causing payment errors.
And now, a summarization summary
To summarize 🙂, LLMs got really good at producing text summaries that read well. This allowed us to focus on what a summary created for a particular purpose must look like. Looking at the problem of summarizing customer support conversations as a question-driven briefing by a capable AI assistant is the key to high quality conversation summaries for CX professionals.
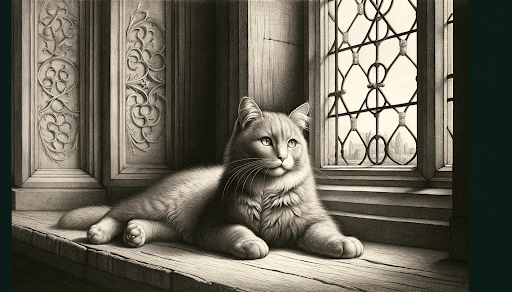