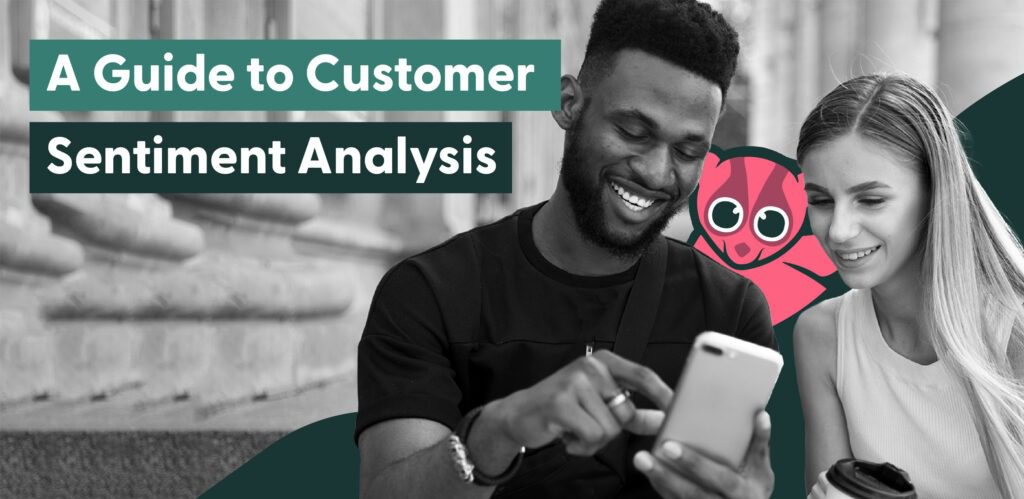
Your customer support team is the face of your business. If they don’t get their customer service interactions right, you may lose customers.
In fact, 96% of customers will leave a brand due to poor customer service. Can you afford this kind of churn? Most businesses can’t.
The good news is that 70% of unhappy customers will stay with a company if customer service resolves their problems.
But how do you get this right?
One approach is customer sentiment analysis, which can help instantly boost your customer service team’s performance.
However, manually analyzing customer sentiment can be time-consuming and makes it difficult to get accurate insights. That’s why we recommend using a conversational intelligence platform to manage this for you.
In this guide, we unpack customer sentiment analysis, including how to measure this important metric and how it can be used to ensure customer satisfaction.
Contents
What Is Customer Sentiment Analysis?
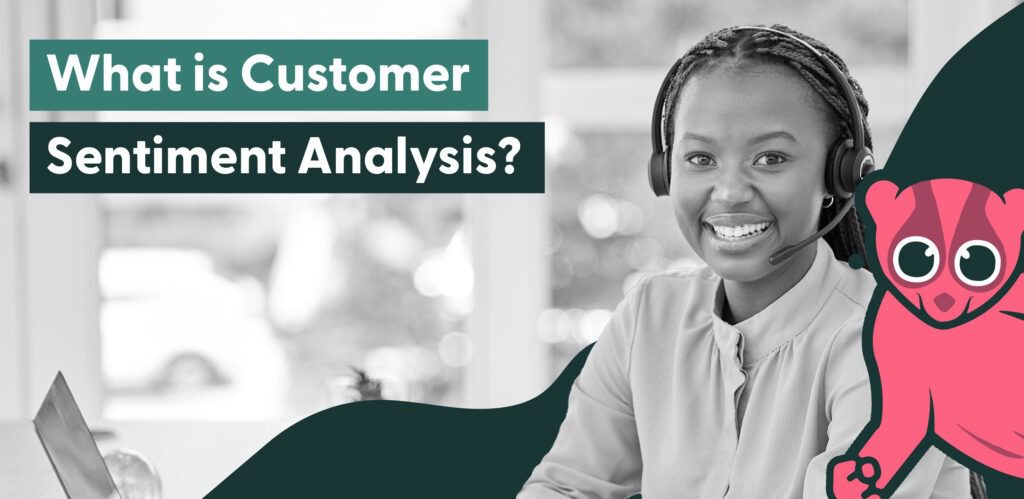
This article discusses sentiment analysis in the context of customer support. Sentiment analysis involves determining your customers’ attitudes, emotions, and opinions when dealing with your support team.
For example, if a customer contacts your support center because they are dissatisfied with your product, this would reflect a negative sentiment toward your brand. However, if your support agent is able to resolve the issue efficiently, the conversation may end with a positive sentiment.
The main objective of sentiment analysis is understanding how your customers feel—positive, negative, or neutral—about your business.
Analyzing customer sentiment can help your support agents quickly assess the urgency of a customer issue. This empowers your agents to turn negative sentiment into positive results.
It is an efficient way to identify emerging issues with your product or service. This could be related to the product itself, a delivery problem, or an unsatisfactory support interaction.
You can also track customer sentiment over time. For example, if you’ve recently changed your product and see an increase in positive sentiment over the next month, this may indicate the changes you made were successful.
However, if there’s no measurable difference in your customer sentiment after having made a change to your product, there may still be room to improve.
🤔 Is customer sentiment analysis different from CSAT?
While your customer satisfaction score (CSAT) is a relevant metric to assess customer experience (CX), sentiment analysis gives you a broader picture.
CSAT is derived from asking customers specific questions about their experience with your business. The purpose is to measure their satisfaction level at a certain time.
For example, after a support interaction, customers may be directed to an automated survey where they can rate their experience. This is used to assign a CSAT score.
However, this score fails to capture the full spectrum of customer emotions and opinions.
Additionally, the results of your CSAT survey may be biased, as not everyone will complete the survey. Your insights will, therefore, only be based on a small sample of conversations.
🤔 Is customer sentiment analysis different from NPS?
Your net promoter score (NPS) measures customer loyalty and their likelihood of recommending your brand to others.
It is usually determined through a single question on a scale of zero to 10: How likely are you to recommend our company to a friend or colleague?
This question can be asked while a customer is still on a call or after the interaction via email or text.
Customers are then categorized as:
- Promoters (9 to 10)
- Passives (7 to 8)
- Detractors (0 to 6)
NPS is calculated by subtracting detractors from promoters.
However, this is where NPS ends. It doesn’t provide clear insight or real-time feedback that you can use to train your support agents or make data-driven decisions within your business.
Why Is Sentiment Analysis Difficult?
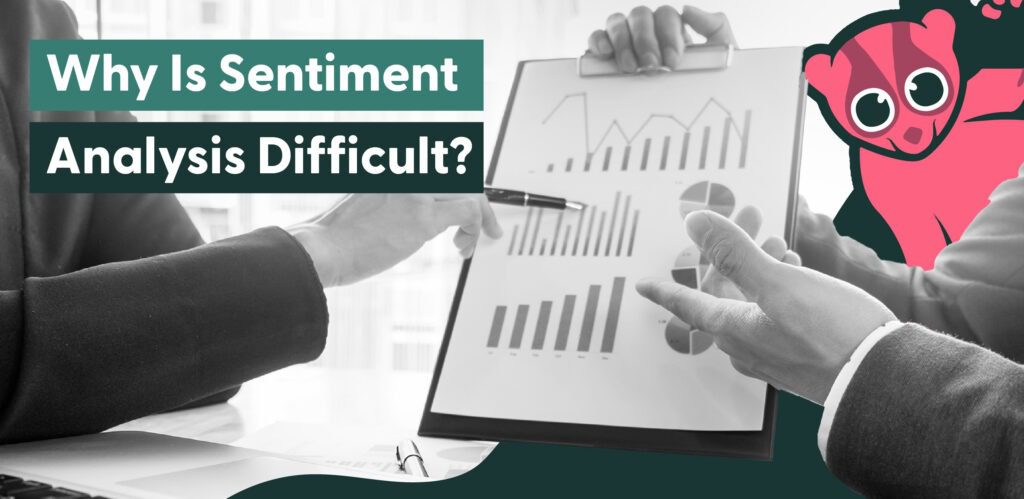
Here are some of the customer sentiment analysis challenges:
➡️ It’s time-consuming
Sentiment analysis involves a lot of work: studying interactions, recording results, analyzing data, and drawing conclusions. It also includes tagging the reason behind the customer contact and if there is anything the agent could’ve done better. If performed manually, it can take up a lot of the QA manager’s time.
For example, manual sentiment analysis requires Customer Service and QA managers to sift through hundreds (if not thousands) of call, chat, and email transcripts. They do this to determine how customers felt during and after support interactions.
This manual work takes up valuable time and resources that could be spent on improving your QA process and better supporting your agents.
➡️ You often rely on incomplete data
Because sentiment analysis is so time-consuming, QA managers often don’t have time to study every interaction.
This means they draw conclusions based on a small sample of customer interactions. The result is unreliable data that leads to inaccurate conclusions. This limits the effectiveness of the training programs that the data and analysis informs.
➡️ Calculating sentiment is complex
If you have a manual QA process, you must calculate customer sentiment scores yourself.
This cumbersome process involves subtracting the occurrences of negative feedback from the number of positive sentiments. You then have to divide this by the total number of customer interactions. And the interpretation of positive and negative can be subjective—especially for distributed teams in different regions or countries.
Calculating sentiment is another time-consuming process that prevents QA analysts and management teams from focusing on improving support interactions.
Today, measuring customer sentiment looks different. AI solutions have been developed to calculate sentiment analysis instantly.
➡️ There’s a lack of understanding of human language
Many customer sentiment analysis platforms are designed to search interactions for positive and negative keywords or phrases. The tool then assigns a positive or negative sentiment based on the number and frequency of the terms detected in a conversation.
The problem with this approach is that each conversation has nuances that these platforms don’t understand. Such platforms only provide minimal conversation data and don’t understand subtlety or context if phrases within the conversation don’t match their libraries of terms.
For example, some customer sentiment analysis tools can’t tell how the word “no” is used in an interaction. “No” can be both positive and negative, like if a customer says, “No, it’s not working,” or if they say, “No, I’m all set.”
The first use of the word “no” has a negative sentiment, and the second use of the term has a positive sentiment. Some tools won’t be able to tell the difference, giving you inaccurate data.
Another example is that conversations with a customer service manager will typically be assigned a negative rating because it’s usually the result of an escalation. This data makes it seem like the customer service manager only has negative sentiment in their customer interactions, which might not be the case.
💡 How AI sentiment analysis tools can help
Rather than relying on low-response surveys or combing through hundreds of call and chat transcriptions—you can use customer sentiment detection tools within your quality assurance (QA) process.
Many of these tools use artificial intelligence (AI) and machine learning (ML) to analyze customer conversations and automatically collect data on customer sentiment.
This allows you to collect data from all interactions, giving you access to more customer sentiment data than ever before.
Plus, you can monitor sentiment in real time, enabling your agents to resolve issues proactively.
💡 Top Tip: Review 100% of Conversations for Accuracy
To have an accurate understanding of overall customer sentiment, you need to analyze every customer interaction. However, doing this manually is nearly impossible for QA teams.
Loris collects data from 100% of your interactions and assigns customer sentiment to every sentence of a call or message. This makes it easy for your team to measure and track customer sentiment.
Our data provides insight into the quality of conversations on a large scale for you to analyze.
Seth Levine, Lead Machine Learning Scientist at Loris, says, “There’s a certain trajectory that most conversations go through. The customer comes in, they’re usually dissatisfied. [Agents can] bring it up to neutral or a slightly satisfied level.”
“Since we’ve analyzed so many conversations, we know what that trajectory should look like, and we can tell when it’s not [the right] trajectory. We can also give a rating of the conversational quality (CQ) based on the customer satisfaction level.”
Ways to Use Customer Sentiment
There are two main ways you can measure customer sentiment within your contact center:
💬 In a live conversation
Using customer sentiment analysis, you can view messages as they take place to measure a customer’s sentiment.
AI tools can provide live feedback at critical moments throughout a customer interaction. For example, they might provide a traffic light system to indicate the customer’s emotional state. If the customer is red, i.e., angry or upset, then the customer service agent can de-escalate the situation. This may help agents turn negative sentiments into a positive one.
📈 Post-interaction reviews
With AI-powered QA tools, you can conduct post-conversation reviews that help you understand overall sentiment and performance—on an individual, team, and organizational level—and pinpoint common issues.
A crucial part of a post-interaction review is to provide better coaching for your agents to help them resolve issues effectively.
The Benefits of Using Customer Sentiment Analysis
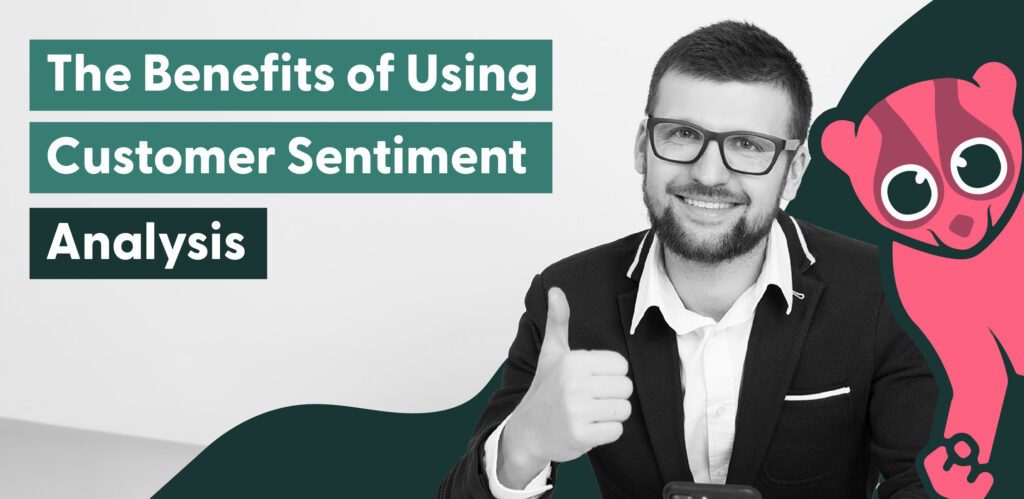
Sentiment analysis gives you better insight into your customers than CSAT and NPS by providing feedback directly from customer language in everyday conversations. But there are some other ways that analyzing customer sentiment is useful:
✅ Predict problems during live interactions and avoid escalations
The best sentiment analysis tools track customer sentiment during live support conversations.
This helps your agents identify the direction the interaction is taking. Sentiment analysis tools can provide your customer service teams with real-time guidance during a live interaction on how they can resolve issues.
Doing this also reduces the number of customer interactions being escalated to management teams.
With Loris CoPilot, AI guides your customer service team through support conversations by giving agents the right responses to customer inquiries and live customer sentiment so they have the right answers at the right time.
💡 A Word from Our Experts:
When speaking about Loris CoPilot, our Head of Marketing, Ciaran Doyle, says, “If you know customers are having a problem and you want to help your agents deal with it better, wouldn’t you want them to be able to respond in the right way? In doing this, you have fewer issues because you’re giving agents what they need in the moment.”
✅ Understand where you can improve your offerings
Analyzing customer sentiment helps you understand how customers feel about your product or service so you know what’s working (and what’s not).
Customer sentiment analysis lets you discover common issues with your offering and where to improve. You can even tie customer sentiment to types of conversations, so you can see if there is a relationship between certain products and low customer sentiment. You can also measure how effective your improvements are by monitoring customer sentiment over time.
✅ Predict CX issues in real time
Some customer sentiment analysis tools allow you to track a customer’s sentiment in live customer conversations. This can suggest ways to improve sentiment based on what the customer says. For example, it may prompt the agent to reassure frustrated customers or provide guidance to those with product questions.
✅ Improve overall CX
Customer sentiment analysis tools not only give you an overview of customer sentiment for each conversation, but also your entire contact center. They help you understand common ways that support interactions go wrong. You can improve on these points to provide a better customer experience (CX).
Let’s say you notice customer sentiment dips when agents provide support for a certain aspect of your product. This may indicate they need more training on it.
✅ Understand agent performance
Up to 80% of customers say agents have the biggest impact on their experience with a business.
Customer sentiment analysis helps you uncover where each agent is performing well and where they need coaching to improve.
This is useful for informing training programs—but it’s also useful for agent performance interventions and reviews.
For example, if you see that one agent continuously ends calls on negative sentiment, you can address this with them.
💡 A Word from Our Experts:
When speaking about Loris CoPilot, our Head of Marketing, Ciaran Doyle, says, “If you know customers are having a problem and you want to help your agents deal with it better, wouldn’t you want them to be able to respond in the right way? In doing this, you have fewer issues because you’re giving agents what they need in the moment.”
✅ Deliver informed training
Providing regular training to your customer support agents is crucial if you want to give your customers a seamless experience.
Research has found that customers are more likely to remain loyal to a brand if they experience knowledgeable agents who are friendly and quick to provide assistance.
The insights you get from customer sentiment analysis can help you identify where each agent can improve. It also helps you find common issues you can resolve to elevate your support team.
You can deliver data-driven training to your agents so that they can perform at their best and better represent your brand.
For example, let’s say your customer sentiment analysis reveals that your agents aren’t empathetic enough toward customers. This can help you plan a training session dedicated to correcting this.
✅ Build your reputation
Collectively, businesses in the U.S. lose $1.6 trillion as a result of customers switching to competitors due to poor customer service.
However, providing customers with a seamless support experience will positively impact your brand’s reputation.
Drawing data from every customer interaction gives you a wealth of information. You can use this to spot trends and predict what customers will likely be unhappy about. You can then design your support process to handle these issues effectively.
A Modern Approach to Sentiment Analysis
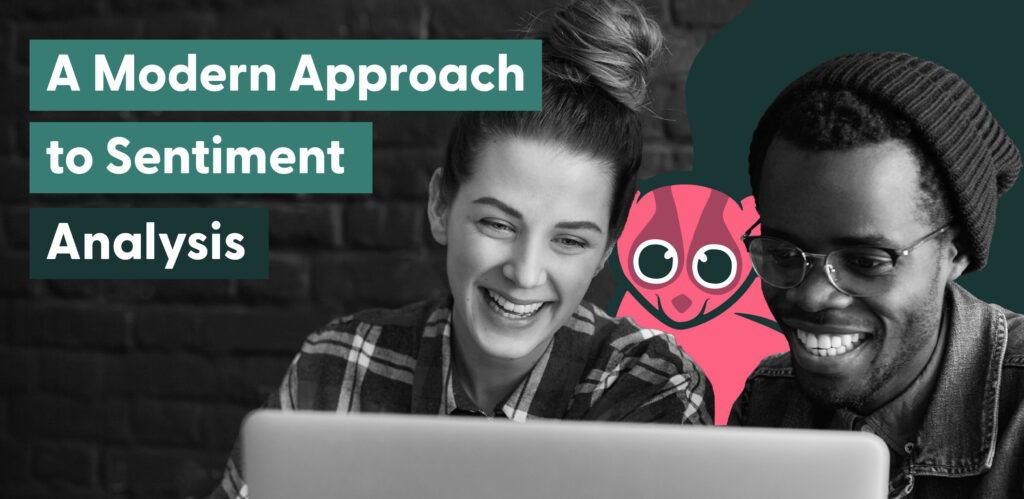
Modern solutions use AI and automation to monitor sentiment in real-time and map it out during a live conversation.
Reviewing hundreds of recorded calls and messages and spending hours monitoring live interactions is now a thing of the past.
Conducting surveys and manually calculating customer sentiment is also no longer necessary.
The Loris conversational intelligence platform includes AI customer sentiment analysis capabilities (and so much more) that go beyond collecting customer data and feedback to analyze sentiment.
“We look at every sentence individually, and we rate the sentiment on a scale of negative two (-2) to positive two (+2),” says Ciaran.
In the conversation view of our dashboard—whether it’s a live conversation or a review—you can see a sentiment map of the conversation in real-time.
“This is also good if you’re a QA analyst analyzing a conversation. You can view the very positive interactions, but, more importantly, you can view the negative ones,” Ciaran explains.
“You don’t have to read through everything. It’s a lot easier,” Ciaran says. “If you know you can only review 15 to 20 conversations a day, you want to make them the right conversations.”
Other Loris features that can help you analyze customer sentiment include:
✔️ Sentiment target classification: Analyze who the customer is directing the sentiment of their response toward, either your agent or brand. Around 70% of customer conversations have sentiment directed toward brands, which helps you differentiate between agent performance issues and overall company or product issues.
✔️ Conversation Quality (CQ) score: This measures the health of your CX by determining how satisfied a customer was and the critical issues that needed to be addressed. This provides a predictive customer satisfaction score without having to conduct a separate CSAT survey.
✔️ Voice interaction analytics: Loris analyzes voice calls for agent efficiency metrics. We use AI models to determine CQ and our live sentiment graph helps you evaluate support calls.
⚠️ The Problem with Some Sentiment Detection Tools
Many customer sentiment tools randomly choose which interactions they analyze.
This approach isn’t representative as it doesn’t give you complete data. As discussed earlier in the article, this means the insights you generate won’t be as accurate. Additionally, keyword-based sentiment tools are flawed as they only reveal data based on an analysis of certain keywords in a conversation rather than providing an overview of all your customer interactions. They often fail to place interactions in context and grasp the nuances of customer interactions.
Boost Your Customer Support Team Performance with Loris
With an AI-powered conversational intelligence platform like Loris, you can analyze all your interactions and pinpoint issues. You can also tailor your feedback to each agent and boost your support team’s overall performance with targeted training, which ensures you have happy customers.
Visit our website to speak to a Loris expert about how our platform can improve your customer sentiment analysis process to get the best results.